Who does what with data
Analytical Roles: Gaining Insights from Data
A good example of an analytical role is that of a data analyst. A data analyst examines sales figures and, based on their findings, advises for example on the assortment of a retail chain. By recognizing patterns and trends in large datasets, the data analyst helps organizations make better-informed decisions. Besides sales analysis, data analysts may also focus on customer behavior, operational processes, or market developments.
IT-Intensive Roles: Building and Connecting Data Systems
On the IT side, there are roles mainly focused on technically managing and connecting data sources. An important example is the role of the data engineer. A data engineer develops and maintains complex infrastructures to ensure that data remains secure, structured, and accessible. Think, for instance, of programming systems that automatically synchronize point-of-sale data with a central database. Thanks to the work of data engineers, organizations can use up-to-date information for analyses, dashboards, and sales forecasts.
Increasing Collaboration Between Roles
The line between analytical and IT-intensive roles is becoming increasingly blurred. Data analysts are working more closely with data engineers, data scientists, and business intelligence specialists. Together, they ensure that data is not only correctly collected and stored but also effectively analyzed. This makes it possible to fully leverage data for strategic and operational purposes.
The Future of Working with Data
In a data-driven world, the demand for professionals in both analytical and technical data roles continues to grow. Companies are investing in modern data platforms and are looking for employees who not only have technical skills but are also able to create valuable insights from data. Working with data has thus become an essential part of the digital transformation of organizations.
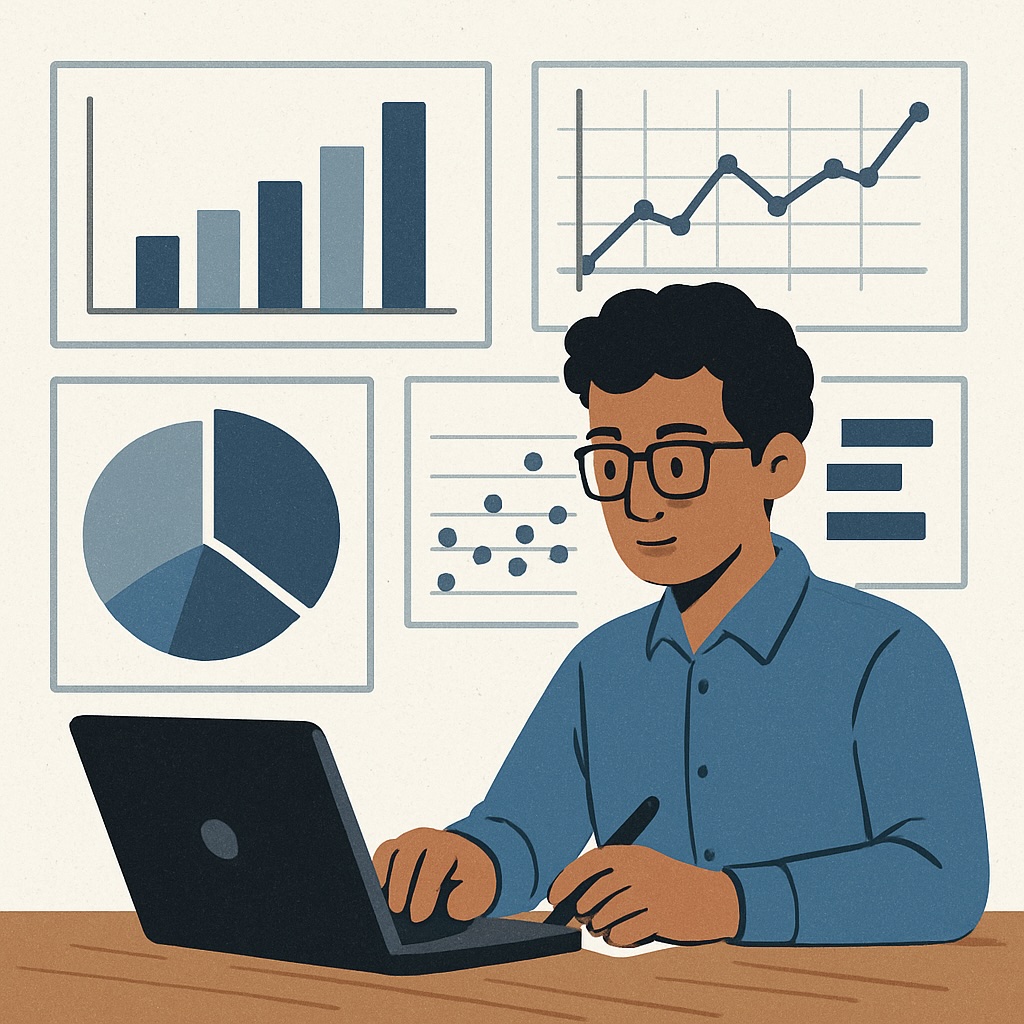
Classical data
Classic Data: Working at Different Scales
The major complicating factor in the world of data continues to be scale. Scale not only determines the technical challenges but also the way work is organized. Within the world of classic data, this plays an important role.
A classic data analyst is generally responsible for the entire data process: from initial data collection, cleaning and structuring the data, to the analysis and delivery of the final product, such as a report or dashboard.
In traditional environments, this process may revolve around relatively small datasets consisting of a few hundred or thousand data points. Think of market research, customer satisfaction surveys, or financial reporting within SMEs.
At the same time, a classic data analyst can also work with datasets containing millions of data points, for example at large organizations in sectors such as telecom, financial services, or healthcare. Here, the volumes are much larger, but the core of the work remains the same: extracting insights from data and translating them into valuable management information.
The Changing Role of the Data Analyst
The role of the data analyst is constantly evolving. Due to the growing amount of available data and the rise of advanced analytics techniques such as machine learning and artificial intelligence, the focus is increasingly shifting towards specialization.
However, there will always be a need for professionals who can master the entire process: from raw data to actionable insights. Especially in smaller organizations or departments without large data science teams, classic data analysts are indispensable. They combine technical skills with analytical thinking and know how to clearly present complex data to non-technical stakeholders.
Why Scale and Context Are Essential
Working with classic data requires an understanding of scale and context. A dataset of a thousand rows can be just as complex in one situation as a dataset of a million rows in another.
Important factors such as data quality, consistency, and the purpose of the analysis play a crucial role. Understanding this dynamic is essential for any data analyst working with classic data.
The Future of Classic Data
Despite the growing popularity of big data and advanced analytics tools, classic data remains a foundation within many organizations. The ability to quickly and efficiently extract insights from traditional datasets remains highly valuable.
Organizations are still looking for data analysts who not only have technical knowledge but also a broad perspective, attention to detail, and the ability to translate data into understandable, action-oriented information.
In short, the classic data analyst – now and in the future – plays a key role in successfully leveraging data within any organization.
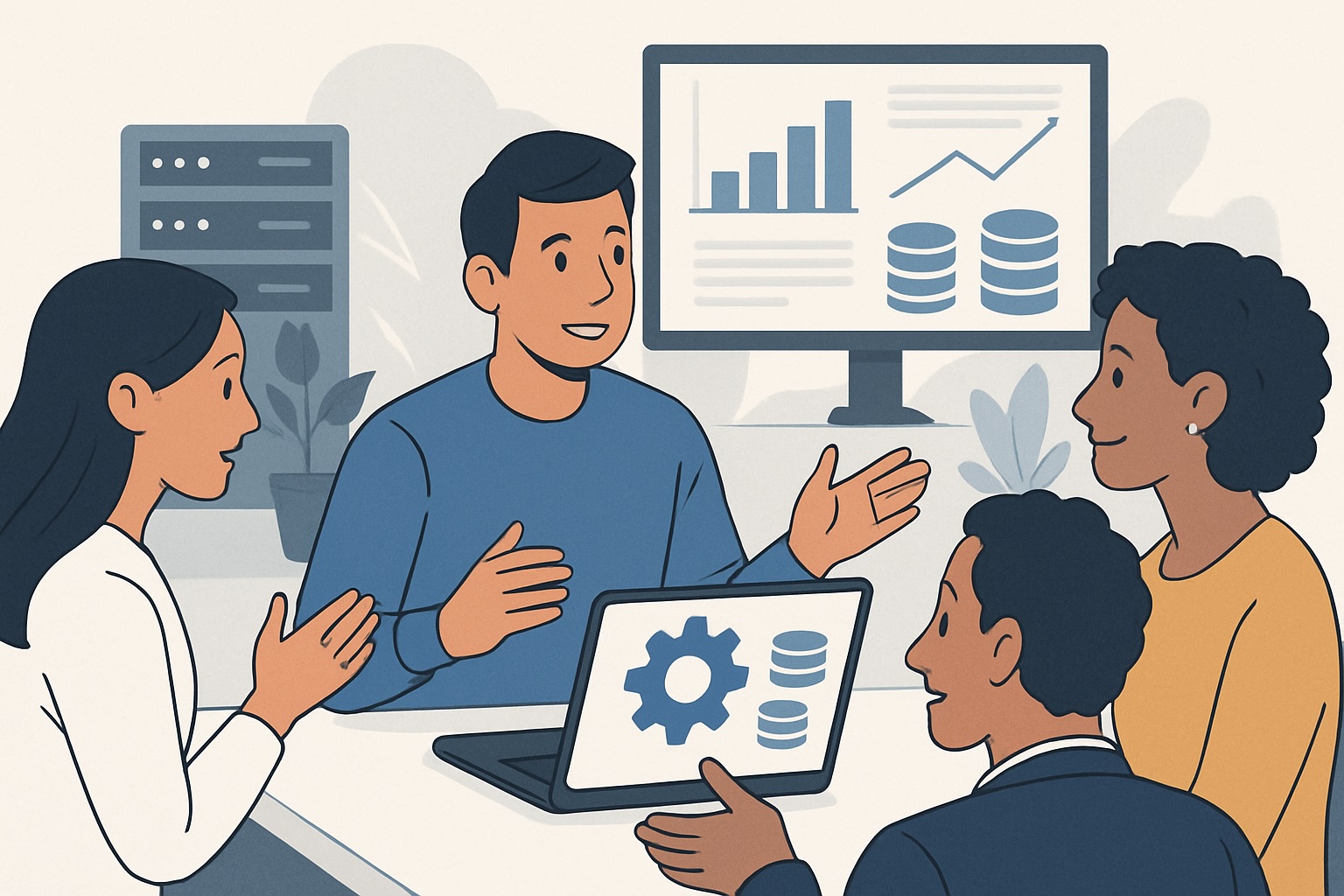
Big Data
What is Big Data?
We speak of big data when datasets consist of millions or even billions of data points, often continuously expanding. This immense volume of data exceeds the capabilities of traditional data processing tools to effectively handle, analyze, and manage. Big data forms the backbone of modern data-driven organizations and offers unprecedented opportunities for deep insights and innovation.
Why is Big Data so important?
In a world where data continues to grow exponentially, big data is essential for organizations that want to remain competitive. By making smart use of big data, companies can predict customer behavior, optimize internal processes, discover new market opportunities, and manage risks more effectively. Big data makes it possible to see patterns and connections that would simply remain hidden with smaller datasets.
The challenges of Big Data
When dealing with such enormous amounts of data, the size and complexity of the various steps from data collection to insights also increase. Traditional methods fall short, and therefore it is necessary to:
- Break down the data analysis process into manageable steps;
- Involve specialists, such as data engineers, data scientists, and data analysts;
- Use advanced technologies such as artificial intelligence and machine learning to gain insights.
Technology behind Big Data
The enormous computing power needed to process big data usually cannot be provided by standard computers. Instead, companies make use of:
- Supercomputers: Machines capable of performing massive amounts of calculations per second.
- Cloud services: Flexible solutions where computing power and storage capacity can be scaled up as needed without heavy investments in physical infrastructure.
- Decentralized systems: Such as Hadoop and Spark, which allow data to be distributed across multiple servers and processed in parallel.
The future of Big Data
Big data is evolving rapidly. With emerging technologies like quantum computing, real-time data analysis, and advanced AI models, the possibilities are only increasing. Organizations that invest in big data strategies now are building a foundation for sustainable growth, innovation, and competitive advantage.
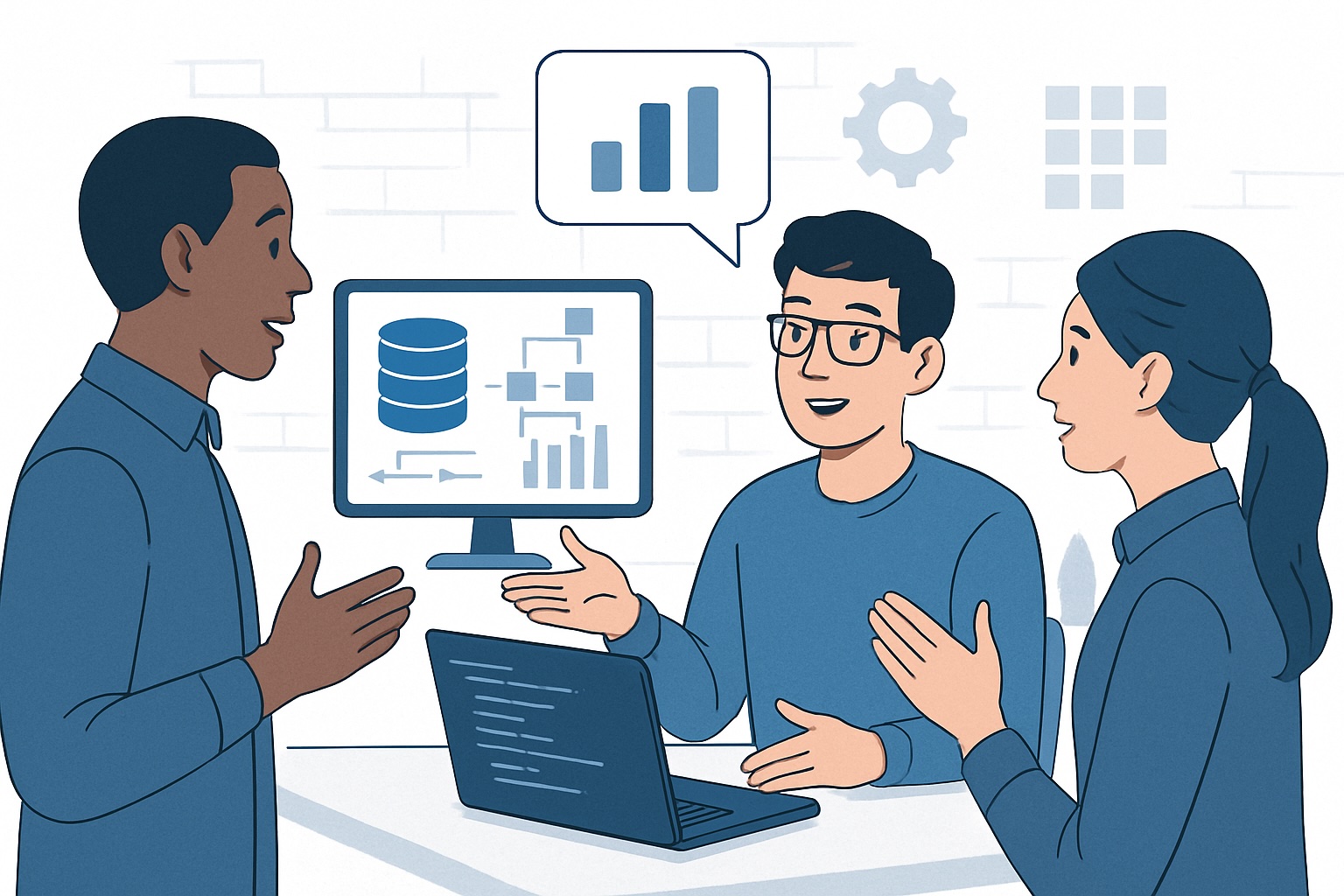
Combinations of roles
Combinations of Roles in Data and Analytics
In this career guide, we discuss various roles within data and analytics. Of course, these roles are somewhat generalized. In practice, we see that the boundaries between functions often blur. More and more role combinations are emerging, where professionals combine multiple specializations to create even more value for organizations.
Shifting Boundaries Between Functions
In the business world, roles are rarely black and white. Many professionals combine knowledge and skills from multiple disciplines. This creates a dynamic work environment where flexibility and a broad skill set are more important than ever.
Examples of Combined Roles
A good example of this is data scientists who also take on part of the data engineering tasks. For instance, they not only build models but also ensure the right data flows and infrastructure to allow these models to perform optimally.
Another example is data analysts who increasingly work with advanced AI tools for tasks such as text analysis and predictions. They use techniques like linear regression models to uncover complex patterns in data.
Why These Combinations Are Important
Combining different skills not only makes professionals more versatile but also more attractive to employers. Companies are increasingly looking for employees who can fulfill multiple roles and adapt quickly to new technologies and methodologies.
By mastering multiple disciplines, you can develop better solutions, collaborate more effectively with different teams, and respond more quickly to changes in the market. Especially in the world of data and analytics, where innovation and speed are crucial, this flexibility is highly valuable.
Future of Combined Roles
The trend toward combined roles will continue to strengthen in the coming years. The demand for professionals who can bridge the gaps between disciplines keeps growing. Think of data engineers with knowledge of machine learning, data scientists with experience in software development, or business analysts who can model complex data.
By continually broadening and deepening your skills, you not only enhance your career prospects but also ensure you remain relevant in a rapidly changing job market.
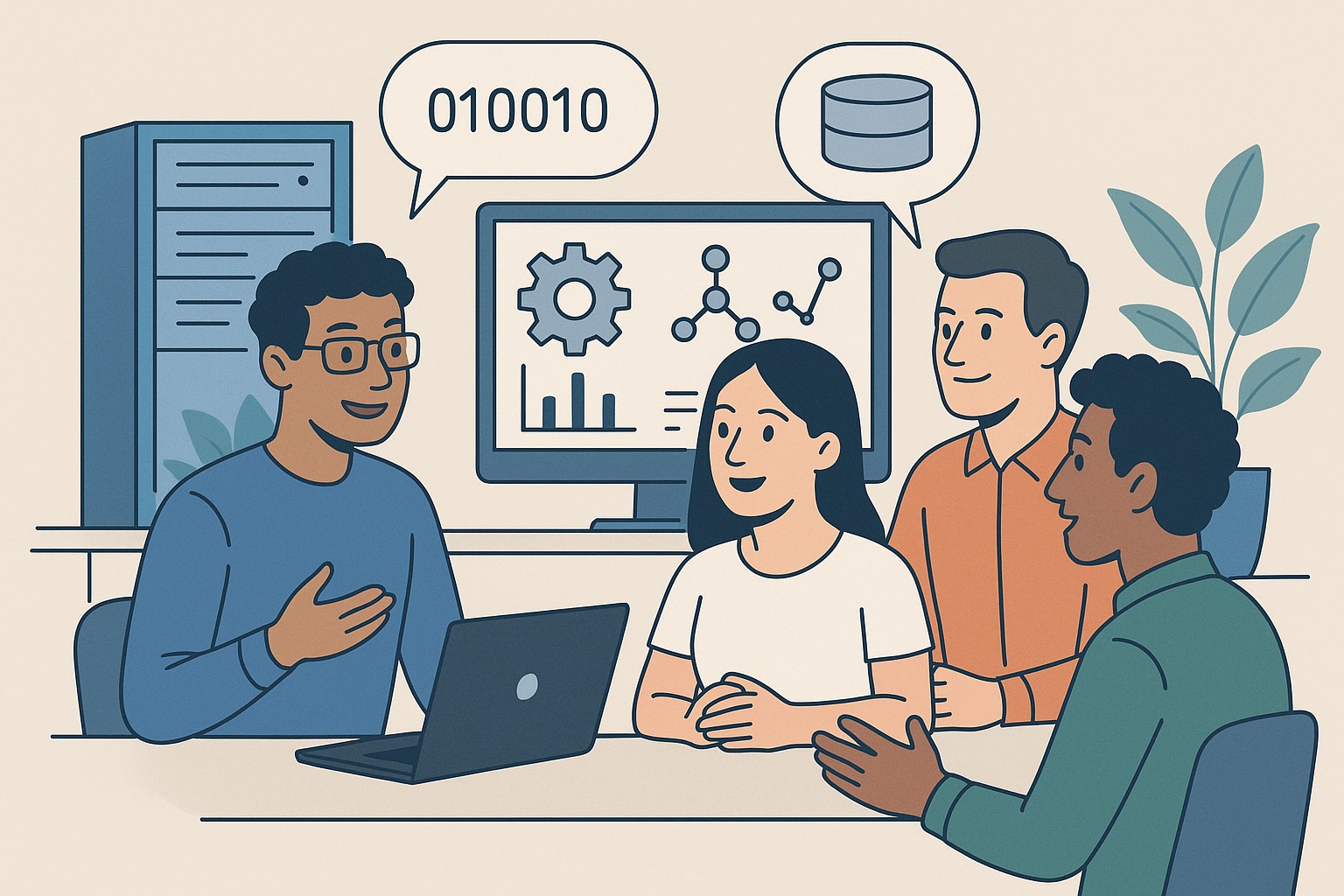
Vacancies for data and analytics specialists
View here all current vacancies on DataJobs.nl
Looking for data and analytics specialists?
For a small fee, you can easily post your job vacancies on our platform and reach our large, relevant network of data and analytics specialists. Applicants respond directly to you, without intermediaries.
On DataJobs.nl, we bring supply and demand in the data and analytics job market directly together—without intermediaries. You won't find vacancies from recruitment agencies on our site. Visitors can view all vacancies for free and without an account, and apply directly.
View the options for posting vacancies here. Questions? Contact us!
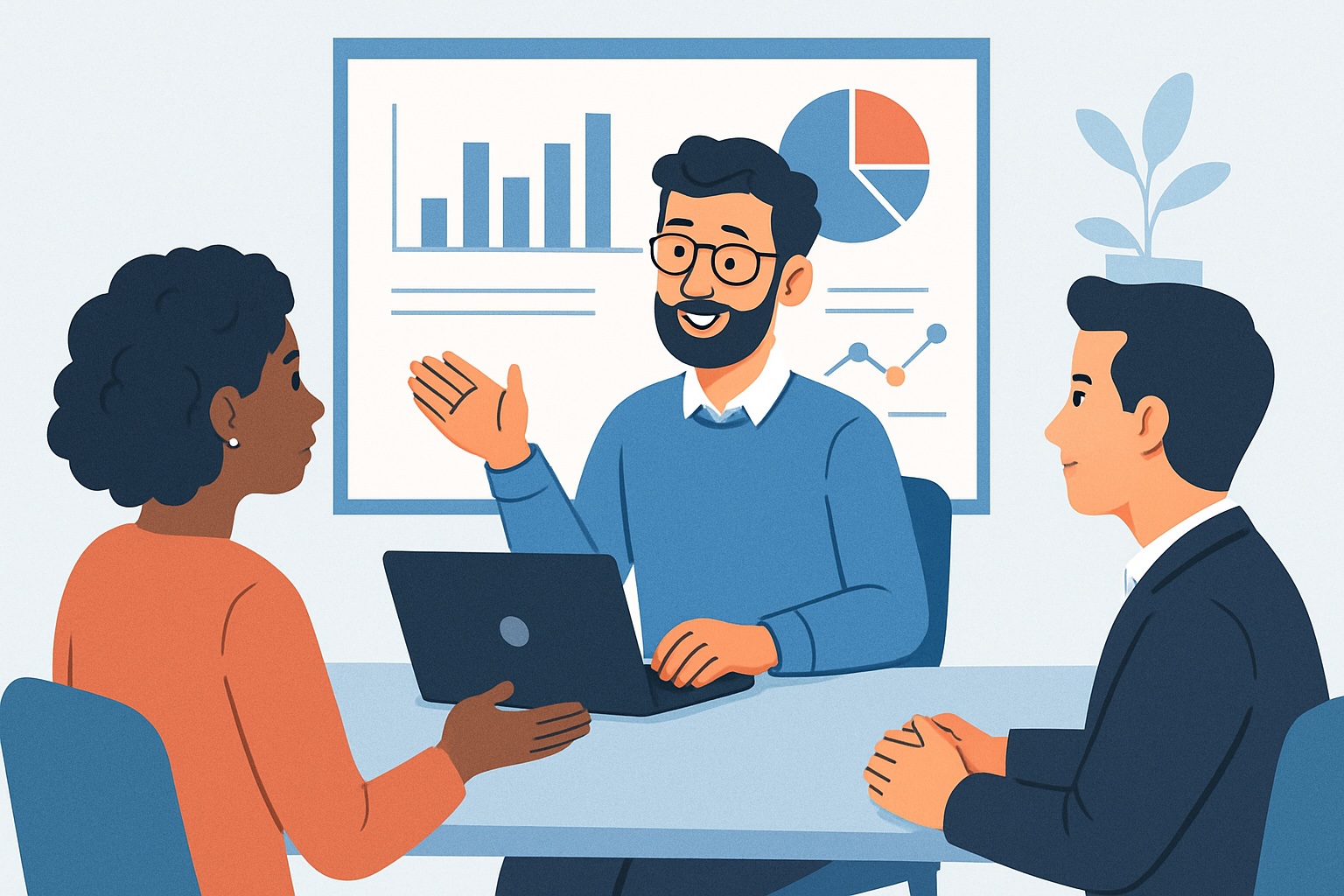